Nature News recently highlighted research by Nathan Ahlgren, assistant professor of biology at Clark University, and his collaborators at the University of Southern California.
The March 19 article, titled “Machine learning spots treasure trove of elusive viruses: Artificial intelligence could speed up metagenomic studies that look for species unknown to science,” focuses on research that Ahlgren began as a postdoctoral research associate in USC’s Department of Biological Sciences, working with Jie Ren, then a graduate student and now a postdoctoral computational biologist at USC.
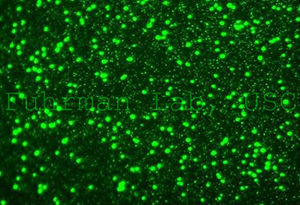
Ahlgren is continuing this research as part of a four-year, $1.5 million National Institutes of Health grant, titled “Computational Studies of Virus-host Interactions Using Metagenomics Data and Applications,” with his co-principal investigator, Fengzhu Sun, professor of biological sciences and mathematics, and his former adviser, Jed Fuhrman, professor of biological sciences, both of USC.“This grant involves developing statistical and computational tools to identify and characterize new viruses that could help us better understand human health, especially viruses that infect bacteria in our intestines,” Ahlgren says.
The Nature News article describes how the USC research team – which included Ahlgren – used algorithms in machine learning, a type of artificial intelligence, to identify previously unknown species of viruses, separate from the bacteria that are also present with viruses.
“In any setting, there will be bacteria, and viruses infecting those bacteria, so when we get DNA sequences from a sample, we get sequences of bacteria and viruses at the same time. We’re trying to distinguish the two,” Ahlgren explains. “We built a machine learning tool that distinguishes these DNA sequences – it’s a kind of categorizing tool.”
“Previously, people had no method to study viruses well,” Ren tells Nature News.
The Nature News report references a 2017 Microbiome journal paper by Ren, Ahlgren, and three other scientists, titled “VirFinder: a novel k-mer based tool for identifying viral sequences from assembled metagenomic data.“